AI Inferences vs Realtime Trading Risk
If overnight and real-time risk can share the same resources and scheduler, why can’t AI training and inference workloads?
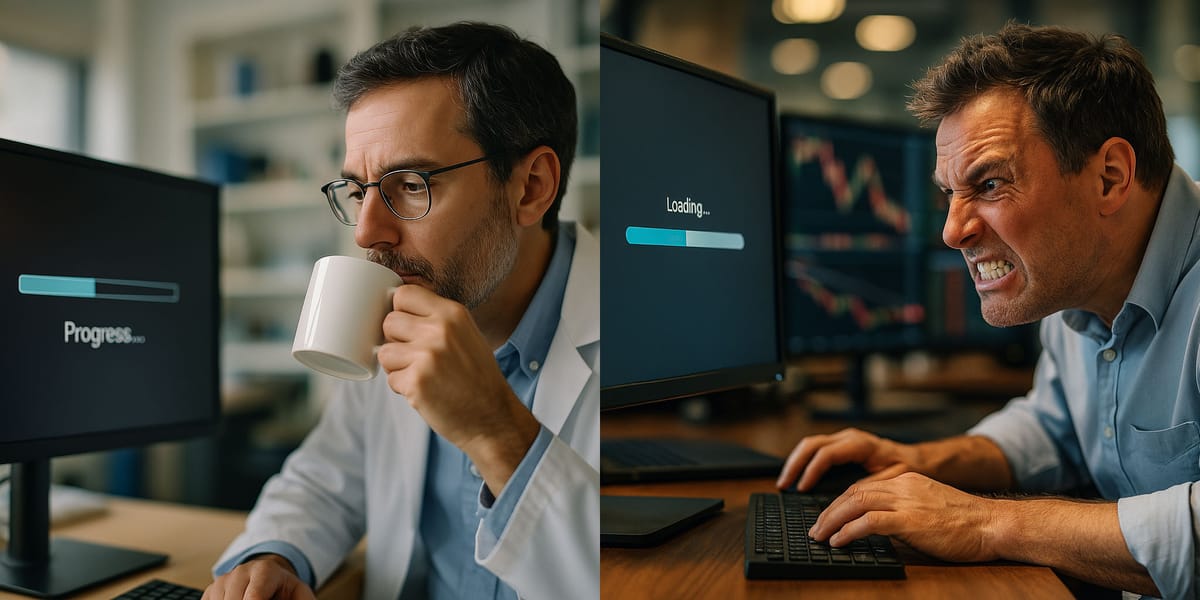
I have a question for the AI Infrastructure readers amongst you.
If I’ve understood this correctly, your training workloads typically use Slurm but your inference workloads will often use something else, usually Kubernetes based. Why?
Splitting your resources into multiple capacity pools, which I assume you must have to do for the above to be true, will only lead to lower utilisation. Why wouldn’t you use the same tech stack to drive your GPUs?
I guess it might be due to the difference in required response times, with inference requiring an immediate answer? But that’s not a new problem.
Within financial services we’ve been using the same compute resources and tech stack (an HPC scheduler) to service not only batched risk operations but also real time trading risk and end of day PnL. Trust me, the irate trader who owns and paid for the GPUs who closing his books at the end of a bad day is going to get upset a lot more quickly than even your $200/month ChatGPT customer!
So, if we can do it that scenario, why can’t (or don’t) AI companies do it for training vs inference? Is this evidence of not invented here syndrome and HPC being too much of a dinosaur for the AI cool kids to consider using it? Or are there genuine reasons why it doesn’t work?